Accurate molecular force fields are of paramount importance for the efficient implementation of molecular dynamics techniques at large scales. In the last decade, machine learning methods have demonstrated impressive performances in predicting accurate values for energy and forces when trained on finite-size ensembles generated with theoretical techniques. At the same time, quantum computers have recently started to offer new viable computational paradigms to tackle such problems. In this work, the authors design a quantum neural network architecture to predict energy and forces and apply it successfully to different molecules of growing complexity. In terms of their effective dimension, the quantum models exhibit better expressivity with respect to classical counterparts and can reach competitive performances, thus pointing towards potential quantum advantages in natural science applications via quantum machine learning.
Quantum neural networks force fields generation
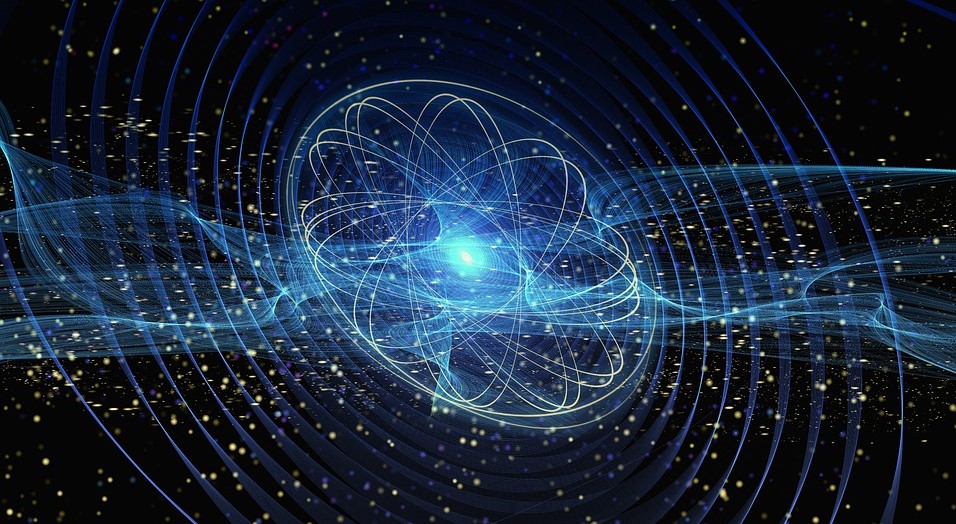
Attachment | Size |
---|---|
Quantum neural networks force fields generation.pdf | 1.69 MB |