The initial wide adoption of Quantum Computing will happen through the integration with large classical systems. Leading technology companies are developing or already providing to the public tools for the orchestration of hybrid computing (for example IBM Quantum Serverless). At the same time, the majority of the algorithms studied today use QC as accelerators for specific tasks embedded in broader classical implementations, variational algorithms being a notable example. In this context, several countries, in Europe in particular, are investing in the integration of Quantum Computing and classical HPC technologies (EuroHPC+EuroQCS). It is important for CERN, as a major actor in the High Energy Physics community, not only to take part in this strategy, but to play a leading and coordinating role to make sure the requirements of the HEP community at large are taken into account while providing compelling use cases for co-development of infrastructures and algorithms. CERN is at the heart of the global collaboration which has been at the base of all the LHC scientific results, the Worldwide LHC Grid. Continuing this leading role in the field of hybrid computing means, today, pursuing development toward exascale infrastructures of increasingly heterogeneous systems including non-Von Neumann architectures, ranging from GPUs to more exotic DL-dedicated ASICS, to tensor streaming technologies, and Quantum Computers.
This Centre of Competence will promote a comprehensive approach toward the construction of a hybrid distributed computing infrastructure, leveraging CERN historical expertise on distributed computing, in collaboration with quantum technology experts in industry, academia, and HPC centres. In this context, in particular, it will leverage the initial links that are being built by the QTI Phase 1, with the HPC infrastructure in the Member States both at a strategic and technical level. Examples include the Barcelona Supercomputing Center (with ongoing discussions with Quantum Spain and Qilimanjaro Quantum Tech), Cineca and its Quantum Computing Lab, the Julich Supercomputing Center and the Leibniz Supercomputing Center with which a join research project is already in place. This Centre of Competence will be implemented using an end-to-end strategy including infrastructure design, the definition and optimisation of hybrid algorithms and the implementation of prototype applications in the field of High Energy Physics.
Physics Theories Simulation
Quantum field theories, by their nature, are rife with infinities. To connect theoretical predictions from QFT calculations to experimental results, these infinities must be dealt with via a process called regularization and renormalization. The only known generic method for doing so non-perturbatively is by implementing the field theory on a lattice, i.e. by discretizing space-time. The dynamics of the discretized theory is then simulated on powerful supercomputers.
Lattice simulations currently provide the only ab-initio method for extracting information about low-energy quantum chromodynamics and nuclear physics, with quantifiable errors. Current lattice QCD simulations successfully predict light hadron masses, scattering parameters for certain few-body scattering events, and the spectrum of several light hadrons. However, due to the limitations of Monte Carlo importance sampling, there are many questions that cannot be addressed with these tools, including the structure of the QCD phase diagram, particularly for large nuclear density, the real-time behaviour of quark-gluon plasmas, and even the masses of any but the lightest hadrons and nuclei.
As the systems under interest are intrinsically quantum, it stands to reason to simulate them on a quantum device. One of our goals is therefore the development of quantum algorithms for lattice simulations.
The results will benefit also our ability to learn about the properties of high-energy QCD (e.g. the parton showers seen in LHC collisions), which on classical computers also suffers from the intrinsic limitations of Monte Carlo sampling.
Another area taking centre-stage in physics research today is the physics of neutrino oscillation (periodic conversions of one neutrino flavour into another during propagation). And while neutrino oscillations are well understood in the context of terrestrial experiments, their dynamics in extreme environments such as supernova cores still evades a full description using classical algorithms. In these environments, neutrino densities are so high that neutrinos influence each other so that the flavour evolution equations become highly non-linear. As this is intrinsically a quantum mechanical, we aim to develop methods for simulating collective neutrino oscillations on a quantum computer.
Quantum Machine Learning
Quantum machine learning (QML) algorithms are a promising approach to certain problems in high energy physics phenomenology and theory, such as the extraction of parton distribution functions from data. We plan to study variational quantum circuits and novel model architectures, which could significantly benefit from a representation on quantum hardware. The main motivation behind this planned activity is to identify the potential benefits of QML in the context of high-energy physics in terms of performance, precision, accuracy, and power consumption.
We will target applications on near-term quantum devices as well as advanced procedures for future, full-fledged universal quantum computers. Research activities will include the development of hybrid classical-quantum models based on variational quantum circuit optimization and data re-uploading techniques, as well as the identification of new hardware designs which may increase QML performance. An important goal of this activity is the collaborative development of open-source quantum machine learning libraries designed for high-energy physics applications.
Objectives
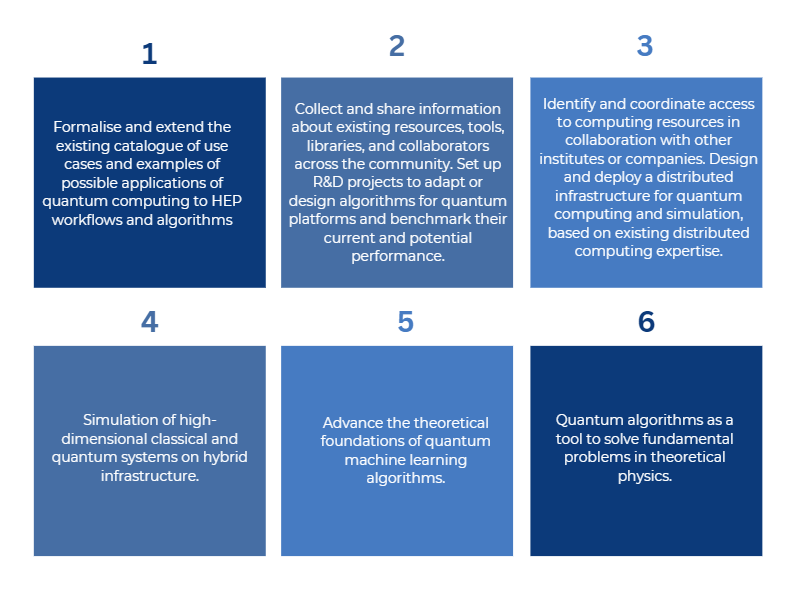
Current and future activities
In HEP, data is generated from a fundamentally quantum process. Even after measurement, quantum correlations between the produced particles can be probed by, e.g., studying the angular distribution of their momenta. The angular correlations between the measured momenta of the particles are a direct consequence of the underlying laws encoded in the amplitude, or equivalently in HEP jargon, the matrix element of the physical process. Theoretically, these amplitudes are computed in the context of the Standard Model of Particle Physics using Quantum Field Theory techniques. The common assumption is that, when designed accordingly, quantum models will be able to naturally deduce these inherently quantum correlations in the data leading to a higher model performance with respect to classical models.
Quantum machine learning could become among the most exciting applications of quantum technologies. However, machine learning algorithms are designed to analyse large amounts of data and they can be considerably different from commonly studied computational tasks. In a NISQ perspective, the challenge related to data and problem dimensionality is important, at the same time, the definition of a quantum advantage for QML approaches can be redefined beyond the simple acceleration/speed-up. Despite the difficulty related to training a model to convergence in a quantum setup, QML could exhibit higher representational power with respect to classical models, and therefore present an advantage in terms of training sample size and/or final accuracy, especially in particularly complicated scenarios, such as those related to generative models.
For this reason, several projects have been launched to better understand the application of the quantum approach to machine learning for HEP data processing. Examples include signal/background classification problems (Quantum SVM for Higgs classification, QML algorithms for classification of SUSY events), reinforcement learning for dynamic optimisation of complex systems (Quantum Reinforcement Learning for accelerator beam steering) and quantum generative models for simulation and anomaly detection (Quantum Generative Adversarial Networks for detector simulation, Quantum Generative Models for Earth observation, Quantum Generative Models for ab-initio calculation of lepton-nucleon scattering). In the same context, different projects will investigate the optimisation and adaptation of well-known quantum algorithms, such as Grover’s search, to HEP data processing. As an example, the project Quantum Algorithms for Event Reconstruction at the CMS detector (Q-Track), is underway and focuses on accelerating, through QC, the TICL framework for HGCAL event reconstruction.
With limited qubit counts, connectivity and coherence times of the present quantum computers, quantum circuit optimisation is crucial for making the best use of those devices. In addition to the above algorithmic studies, this activity intends to study novel circuit optimisation protocols and error mitigation strategies to exploit at best near term quantum devices. As an example, the project Quantum Gate Pattern Recognition and Circuit Optimisation has developed an initial protocol, composed of two techniques - pattern recognition of repeated sets of gates and reduction of circuit complexity by identifying computational basis states, that demonstrates a significant gate reduction for a quantum algorithm designed to simulate parton shower processes. Another critical aspect to achieve the goals described by objective C3 is a systematic approach to benchmarking algorithms, platforms and in the future full-stack quantum systems.
This is particularly important given the increasing number of quantum hardware systems and simulators that exist today. They are provided in a variety of open-source, commercial, cloud or on-premises installation mechanisms. The different implementations exploit technologies or architectures that make different algorithms and applications more or less performant, more or less suitable. In this context, the project Automated Benchmarking and Assessment of QUantum Software (ABAQUS) is intended to design and implement an automated algorithm benchmarking system based on a public database of algorithms, configurations, and platforms. The possibility of sharing such information across a broad community of researchers and creating a “crowd-sourced” collaborative platform would ensure a rapid evolution of computer science and technology
Introducing quantum computing technology in HEP workloads requires a fundamental shift in how algorithms and applications are designed and implemented compared to classic software stacks. Easy access to a variety of hardware and software solutions is essential to facilitate the R&D activity. With the project Distributed Quantum Computing Platform, CERN aims at implementing the key aspects outlined by objective C3, namely building a distributed platform of quantum computing simulators and hardware in open collaboration with academic institutes, resources and technology providers; facilitating access to these resources for the CERN and HEP community; contributing to defining and implementing the layers of future full-stack quantum computing services.
Ongoing projects
Fundamental work is done today in the HEP community and in many other scientific research and industrial domains to design efficient, robust training algorithms for different learning networks.
Initial pilot projects have been set up at CERN in collaboration with other HEP institutes worldwide (as part of the CERN openlab quantum-computing programme in the IT Department) on quantum machine learning (QML). These are developing basic prototypes of quantum versions of several algorithms, which are being evaluated by LHC experiments.
Information about some of the ongoing projects can be found below.
The goal of this project is to develop quantum algorithms to help optimise how data is distributed for storage in the Worldwide LHC Computing Grid (WLCG), which consists of 167 computing centres, spread across 42 countries. Initial work focuses on the specific case of the ALICE experiment. We are trying to determine the optimal storage, movement, and access patterns for the data produced by this experiment in quasi-real-time. This would improve resource allocation and usage, thus leading to increased efficiency in the broader data-handling workflow.
The goal of this project is to develop quantum machine-learning algorithms for the analysis of particle collision data from the LHC experiments. The particular example chosen is the identification and classification of supersymmetry signals from the Standard Model background.
The goal of this project is to explore the feasibility of using quantum algorithms to help track the particles produced by collisions in the LHC more efficiently. This is particularly important as the rate of collisions is set to increase dramatically in the coming years.
The collaboration with Cambridge Quantum Computing (CQC) is investigating the advantages and challenges related to the integration of quantum computing into simulation workloads. This work is split into two main areas of R&D: (i) developing quantum generative adversarial networks (GANs) and (ii) testing the performance of quantum random number generators.
This project is investigating the use of quantum support vector machines (QSVMs) for the classification of particle collision events that produce a certain type of decay for the Higgs boson. Specifically, such machines are being used to identify instances where a Higgs boson fluctuates for a very short time into a top quark and a top anti-quark, before decaying into two photons.